THANK YOU FOR SUBSCRIBING

Future-Proofing Data Center Designs with a Dash of Innovation
Kevin Miller, Principal, Digital Management, Aurecon

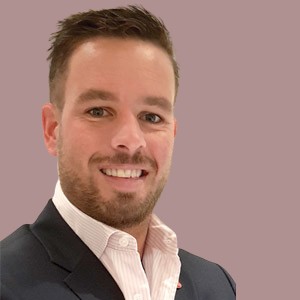
Kevin Miller, Principal, Digital Management, Aurecon
While data centers have, in my 20+ years of experience in designing, building, and operating them, changed in many ways, so much has remained the same. Will the advent of new workloads change that forever? Now, more than ever, the compute loads within them are evolving to a new paradigm driven by requirements for IOT-based data, which in turn will fundamentally change the facilities in which those loads reside.
To understand how this shift is happening, it is worth exploring the evolution of the compute loads over time to bring us to the current day. From the earliest days of the mainframe, traditional data centers relied on defined physical infrastructure dedicated to a specific purpose, with expansion of workloads generally requiring a matched expansion in physical hardware. If you wanted more compute, storage, or networking, you bought more hardware, leading to a expansion of data center area. By today’s standards utilization was exceedingly low, as invariably not all hardware was running at anywhere near full capacity all the time.
Many things have progressed since those physically restrictive days. First we saw virtualization in the early 2000s, which was refined and turbo charged to bring us to the software-defined data center of today. This ushered in an era where a logical computing process was not restricted to a physical piece of hardware but could be moved around the data center in ever more elaborate ways.
These innovations led to significant increases in raw computing per rack. It did not generally, however, translate into huge increases in power and cooling requirements for the facility. This is largely due to the fact that historically load provisioning has been done using a very risk-based approach. In the early days, if you had 10 racks with a peak power draw of 5kW each, you’d likely provision for 50kW of power provision (with a little extra for luck). The fact that each of those servers was almost never at 5kW, didn’t feature into the consciousness of designers because there Kevin Miller 9 July, 2022 was simply no way of knowing what the loads would be, or when. On that one day a year when all servers were running at full throttle, you had to have that power available.
Virtualization made the data centers more dense; more of the time, more of the servers were closer to their maximum. But that maximum didn’t fundamentally change. So you still provisioned your 50kW for your 10 racks, you just used more of it for most of the time.
Yes, density has increased; but generally speaking for all the vast increases in raw compute power for a given rack, the power and cooling requirements have not seen a large step change as a general rule (there are exceptions today just as there were in the mainframe days). Look around and you’d still generally find data centers provisioned for 6-10kW per rack.
Something else which remained constant was the fact that CPUs did all of the real work. These little chips are incredibly good at processing structured data – data in rows and columns – which is how the world ordered its data until recently.
Today, much of the data is unstructured, coming from sources as diverse as a plethora of IOT devices to AI analysis of our sleep patterns to Bitcoin mining. The benefit of this wealth of information is that all of this huge data (both structured and unstructured) holds the key to helping organizations better understand their customers, follow patterns of behaviour to predict future actions, uncover breakthroughs to cure diseases, perform engineering simulations, and make communities safer, among a myriad of other things.
WHILE CPU’S DO HAVE THE CAPABILITIES TO PROCESS TONS OF UNSTRUCTURED DATA, GPUS CAN DO WHAT A CPU TAKES DAYS OR WEEKS TO DO IN A MATTER OF MINUTES
To harness this plethora of data, artificial intelligence and machine learning has emerged, which uses data to train algorithms to find patterns. The problem is, traditional CPUs cannot adequately handle the intensive parallel processing required for machine learning, which is why GPUs are proliferating.
GPUs, which are optimised for parallel computing, were originally developed to improve graphic rendering, but it became clear that GPUs are very useful for any application that needs a lot of data to be crunched quickly, including AI and machine learning, gaming, video editing and content creation, meteorology, and a wide range of scientific applications.
As a result, GPU workload accelerators are fast becoming as necessary to effectively process large data sets (e.g. deep learning algorithms). While CPU’s do have the capabilities to process tons of unstructured data, GPUs can do what a CPU takes days or weeks to do in a matter of minutes. Click on any tech news channel and you’ll hear about GPU shortages owing to blockchain miners buying up the world’s supply. This is because GPUs can uniquely solve these complex computational challenges.
For the data center, with increased requirements for AI-processing, GPUs are becoming ubiquitous. This, in turn, is seeing a requirement for not just increased power and cooling, but for more directed cooling.
A GPU can draw several times more power than a CPU, and has a much higher thermal design point (TDP). This means that it gets hotter in one spot so needs more directed cooling; take a look at any consumer grade GPU and note massive cooling fans (and often water cooling blocks). GPUs now mean that individual chips independently need over 3kW of cooling – some servers pack in over 15GPUs in a single frame. In short, adding a GPU alongside their traditional CPU can triple the density requirement of the server; in these deployments 30kW/rack is not uncommon, nor is the fact that direct-to-chip cooling is becoming almost essential.
The interesting thing about all this is that the way we provision for the data center power and cooling is fundamentally changing, with the data center designers now taking a much greater interest not just in the total loads, but the types of loads.
My advice to anyone looking at a new data center strategy? Get your IT teams in the room with the design teams and strategists early in the process; they need – more than ever – to understand the IT stack and the types of applications which will be running on this stack. Unless it’s with your Friday fish supper, no one likes hot chips.
Weekly Brief
I agree We use cookies on this website to enhance your user experience. By clicking any link on this page you are giving your consent for us to set cookies. More info
Read Also
From Chemical Solutions to Data Center Innovations
Ethics & Compliance in a Digital World: Navigating HCP Engagement in APAC
How AI is Driving Innovation and Customer-Centricity in Insurance
The Impact of Smart Infrastructure on Growing Cities
Transforming Engineering through Data Science
Redefining Risk Management: Strategies for a Safer Future
New Hr Capabilities To Face Evolving Technologies
Strengthening The Compliance Fortress In The Banking Sector
